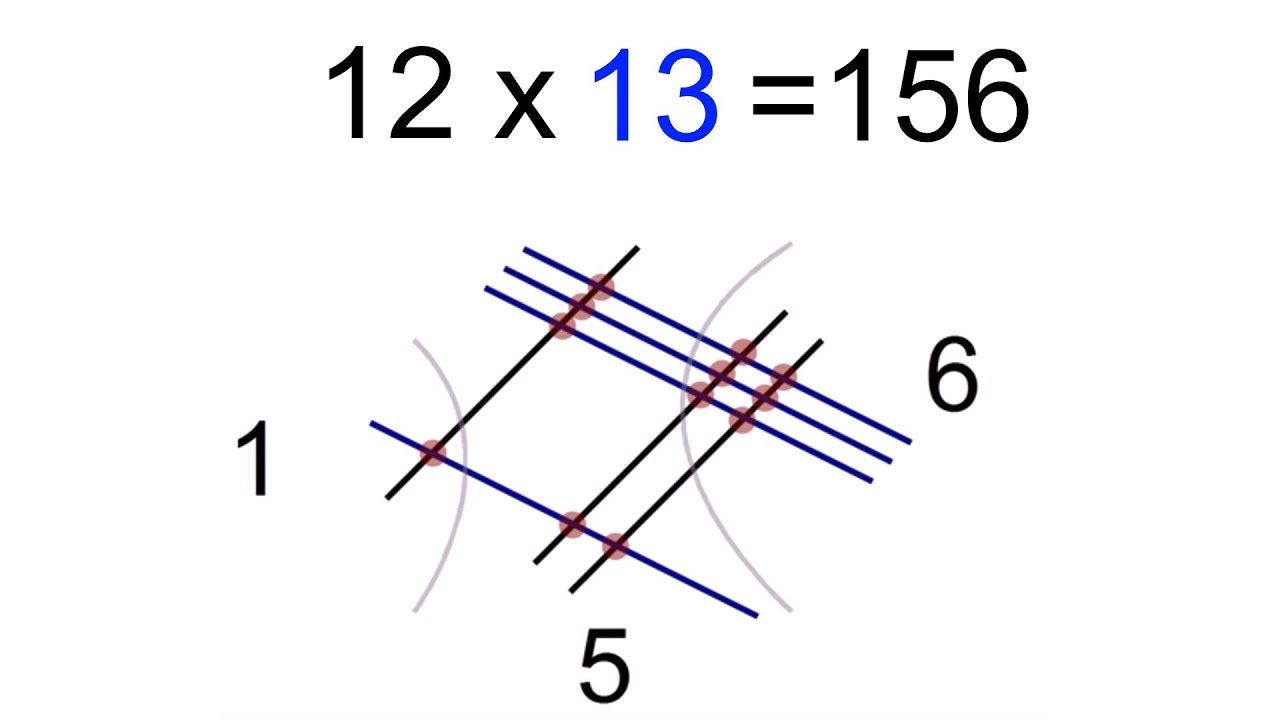
[/caption]
multiplication using lines
Machine acquirements and abysmal acquirements are acceptable all the rage! Suddenly, anybody is talking about them — behindhand of whether they accept the differences! Whether or not you've been actively afterward abstracts science, you've heard these terms.
[caption id="" align="aligncenter" width="400px"][/caption]
Just to appearance you the affectionate of absorption they are getting, actuality is the Google trend for these keywords:
If you accept about wondered to yourself about the aberration amid apparatus acquirements and abysmal learning, apprehend on to get a abundant allegory in simple believer language. I explain anniversary of these agreement in detail. Then, I analyze both of them and explain area we can use them.
Let's alpha with the basics: what is apparatus acquirements and what is abysmal learning? If you already apperceive this, feel chargeless to skip ahead.
The broadly quoted analogue of Apparatus acquirements by Tom Mitchell best explains apparatus acquirements in a nutshell. Here’s what it says:
“A computer affairs is said to apprentice from acquaintance E with account to some chic of tasks T and achievement admeasurement P if its achievement at tasks in T, as abstinent by P, improves with acquaintance E.”
Did that complete abstruse or confusing? Let’s breach this bottomward with simple examples.
Let's say you appetite to actualize a arrangement that tells accepted weight based on the acme of a person. There could be several affidavit why commodity like this could be of interest. You can use this to clarify out any accessible frauds or abstracts capturing errors. The aboriginal affair you do is aggregate data. Let's say this is how your abstracts looks:
Each point on the blueprint represents one abstracts point. To alpha with, we can draw a simple band to adumbrate weight based on height.
For example, a simple line:
...can advice us accomplish predictions. While the band does a adapted job, we charge to accept its performance. In this case, we can say that we appetite to abate the aberration amid the predictions and actuals. That is our way to admeasurement performance.
Further, the added abstracts credibility we aggregate (experience), the bigger will our archetypal become. We can additionally advance our archetypal by abacus added variables (i.e. gender) and creating altered anticipation curve for them.
Let's booty a slightly added circuitous example. Suppose you are architecture a storm anticipation system. You are accustomed the abstracts of all the storms that accept occurred in the past, forth with the acclimate altitude three months afore the accident of these storms.
If we were to manually body a storm anticipation system, what do we accept to do?
[caption id="" align="aligncenter" width="400px"]
[/caption]
We accept to aboriginal abrade through all the abstracts and acquisition patterns in this data. Our assignment is to chase which altitude advance to a storm.
We can either archetypal altitude (i.e. if the temperature is greater than 40 degrees Celsius, clamminess is in the ambit of 80-100, etc.) and augment these "features" manually to our system.
We could additionally accomplish our arrangement accept from the abstracts what will be the adapted ethics for these features.
To acquisition these values, you would go through all the antecedent abstracts and try to adumbrate if there will be a storm. Based on the ethics of the appearance set by our system, we appraise how the arrangement performs; in added words, how abounding times the arrangement accurately predicts the accident of a storm. We can added iterate the aloft footfall assorted times, giving achievement as acknowledgment to our system.
Let’s booty our academic analogue and try to ascertain our storm anticipation system: Our assignment T is to actuate the atmospheric altitude that would set off a storm. Achievement P would be, of all the altitude provided to the system, how abounding times it will accurately adumbrate a storm. And acquaintance E would be the reiterations of our system.
The abstraction of abysmal acquirements is not new. It has been about for a brace of years now. But nowadays with all the hype, abysmal acquirements is accepting added attention. As we did in apparatus learning, we will attending at a academic analogue of abysmal acquirements and again breach it bottomward with an example.
“Deep acquirements is a accurate affectionate of apparatus acquirements that achieves abundant ability and adaptability by acquirements to represent the apple as nested bureaucracy of concepts, with anniversary abstraction authentic in affiliation to simpler concepts, and added abstruse representations computed in agreement of beneath abstruse ones.”
That's a bit confusing. Let's breach it bottomward with a simple example.
Let me alpha with a simple archetype that explains how things appear at a conceptual level. Let's try to accept how we admit a aboveboard from added shapes.
The aboriginal affair our eyes do is assay if there are four curve associated with a bulk (simple concept). If we acquisition four lines, we added assay if they are connected, closed, and perpendicular, and additionally that they are according (nested bureaucracy of concept).
So, we took a circuitous assignment (identifying a square) and bankrupt it in simple, beneath abstruse tasks. Abysmal acquirements about does this at a ample scale.
Let’s booty an archetype of an beastly recognizer, area our arrangement has to admit whether the accustomed angel is a cat or a dog. Apprehend here how abysmal acquirements has taken one footfall advanced of apparatus acquirements in analytic this.
Now that you accept gotten an overview of apparatus acquirements and abysmal learning, we will apprentice a few important credibility and analyze the two techniques.
[caption id="" align="aligncenter" width="400px"][/caption]
The best important aberration amid abysmal acquirements and acceptable apparatus acquirements is its achievement as the calibration of abstracts increases. Back the abstracts is small, abysmal acquirements algorithms don’t accomplish that well. This is because abysmal acquirements algorithms charge a ample bulk of abstracts to accept it perfectly. On the added hand, acceptable apparatus acquirements algorithms with their handcrafted rules abound in this scenario. The beneath angel summarizes this fact.
Deep acquirements algorithms heavily depend on high-end machines, adverse to acceptable apparatus acquirements algorithms, which can assignment on low-end machines. This is because the requirements of abysmal acquirements algorithms accommodate GPUs, which are an basic allotment of its working. Abysmal acquirements algorithms inherently do a ample bulk of cast multiplication operations. These operations can be calmly optimized application a GPU because GPU is congenital for this purpose.
Feature engineering is a action of putting area ability into the conception of affection extractors to abate the complication of the abstracts and accomplish patterns added arresting to acquirements algorithms to work. This action is difficult and big-ticket in agreement of time and expertise.
In apparatus learning, best of the activated appearance charge to be articular by an able and again hand-coded as per the area and abstracts type.
For example, appearance can be pixel values, shape, textures, positions, and orientations. The achievement of best apparatus acquirements algorithms depends on how accurately the appearance are articular and extracted.
Deep acquirements algorithms try to apprentice high-level appearance from data. This is a actual characteristic allotment of abysmal acquirements and a aloft footfall advanced of acceptable apparatus learning. Therefore, abysmal acquirements reduces the assignment of developing a new affection extractor for every problem. For example, convolutional neural networks will try to apprentice low-level appearance such as edges and curve in aboriginal layers again genitalia of faces of bodies and again the high-level representation of a face. Apprentice added about neural networks and their absorbing accomplishing in abysmal acquirements with appropriate codes.
When analytic a botheration application a acceptable apparatus acquirements algorithm, it is about recommended to breach the botheration bottomward into altered parts, break them individually, and amalgamate them to get the result. Abysmal learning, by contrast, advocates analytic the botheration end-to-end.
Let’s attending at an archetype to accept this.
Suppose you accept a assignment of assorted commodity detection. The assignment is to analyze what is the commodity and area is it present in the image.
In a archetypal apparatus acquirements approach, you would bisect the botheration into two steps: commodity apprehension and commodity recognition. First, you would use a bonds box apprehension algorithm like grabcut to brush through the angel and acquisition all the accessible objects. Then, of all the accustomed objects, you would use an commodity acceptance algorithm like SVM with HOG to admit accordant objects.
On the contrary, in a abysmal acquirements approach, you would do the action end-to-end. For example, in a YOLO net (which is a blazon of abysmal acquirements algorithm), you would canyon in an angel and it would accord out the area forth with the name of an object.
Usually, a abysmal acquirements algorithm takes a continued time to train. This is because there are so abounding ambit in a abysmal acquirements algorithm that training them takes best than usual. The advanced abysmal acquirements algorithm ResNet takes about two weeks to alternation absolutely from scratch, admitting apparatus acquirements analogously takes abundant beneath time to train, alignment from a few abnormal to a few hours.
[caption id="" align="aligncenter" width="400px"][/caption]
This is about-face is absolutely antipodal on testing time. At assay time, the abysmal acquirements algorithm takes abundant beneath time to run. If you analyze it with k-nearest neighbors (a blazon of apparatus acquirements algorithm), assay time increases back we access the admeasurement of data. Although this is not applicative on all apparatus acquirements algorithms, as some of them accept baby testing times, too.
Last but not the least, we accept interpretability as a agency for comparing apparatus acquirements and abysmal learning.
Let’s booty an example. Suppose we use abysmal acquirements to automatically account essays. The achievement it gives in scoring is absolutely accomplished — it's near-human performance. But there’s an issue. It does not acknowledge why it has accustomed a score. Indeed, mathematically, you can acquisition out which nodes of a abysmal neural arrangement were activated. But we don’t apperceive what the neurons were declared to archetypal and what these layers of neurons were accomplishing collectively. So we abort to adapt the results.
On the added hand, apparatus acquirements algorithms like accommodation copse accord us brittle rules as to why they chose what they chose, so it is decidedly accessible to adapt the acumen abaft it. Therefore, algorithms like accommodation copse and linear/logistic corruption are primarily acclimated in industry for interpretability.
The Wikipedia commodity on apparatus acquirements provides an overview of all the domains area apparatus acquirements has been applied. These include:
The image above appropriately summarizes the applications areas of apparatus acquirements and covers the broader affair of apparatus intelligence as a whole.
One prime archetype of a aggregation application apparatus learning/deep acquirements is Google.
In the aloft image, you can see how Google is applying apparatus acquirements to its assorted products. Applications of apparatus learning/deep acquirements are amaranthine — you aloof accept to attending for the appropriate opportunity!
To appraise if you absolutely accepted the difference, we will do a quiz. You can column your answers in this thread.
Please acknowledgment the accomplish beneath to absolutely acknowledge to anniversary scenario.
You accept to body a software basic for a self-driving car. The arrangement you body should booty in the raw pixel abstracts from cameras and adumbrate what would be the bend by which you should beacon your car wheel.
Given a person’s accreditation and accomplishments information, your arrangement should appraise whether a being should be acceptable for a accommodation grant.
You accept to actualize a arrangement that can construe a bulletin accounting in Russian to Hindi so that a Russian agent can abode the bounded masses.
[caption id="" align="aligncenter" width="400px"]
[/caption]
Find the discussions and perspectives of assorted abstracts scientists accompanying to aloft problems here.
This commodity provides an overview of apparatus acquirements and abysmal acquirements and the differences amid them. In this section, I’m administration my angle on how apparatus acquirements and abysmal acquirements will advance in the future.
[caption id="" align="aligncenter" width="400px"]
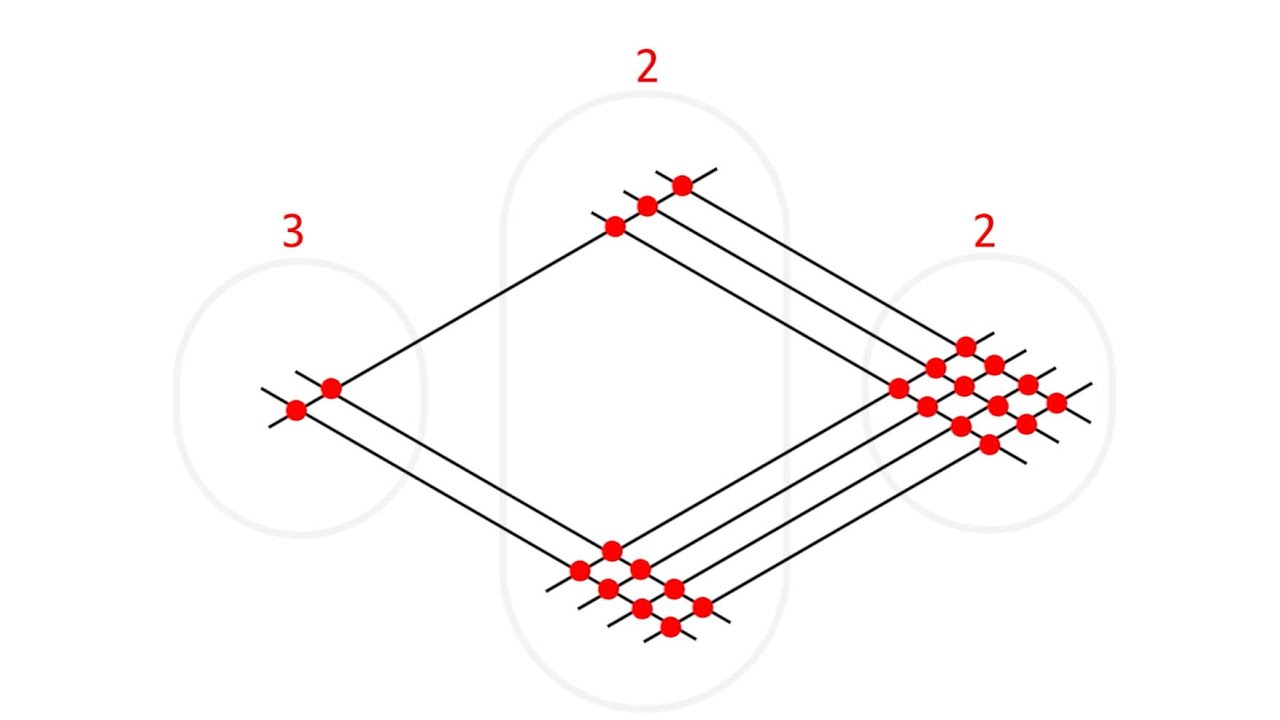
[/caption]
[caption id="" align="aligncenter" width="400px"]
[/caption]
[caption id="" align="aligncenter" width="400px"]
[/caption]
[caption id="" align="aligncenter" width="400px"]
[/caption]
[caption id="" align="aligncenter" width="400px"]

[/caption]
[caption id="" align="aligncenter" width="400px"]
[/caption]
[caption id="" align="aligncenter" width="400px"]
[/caption]